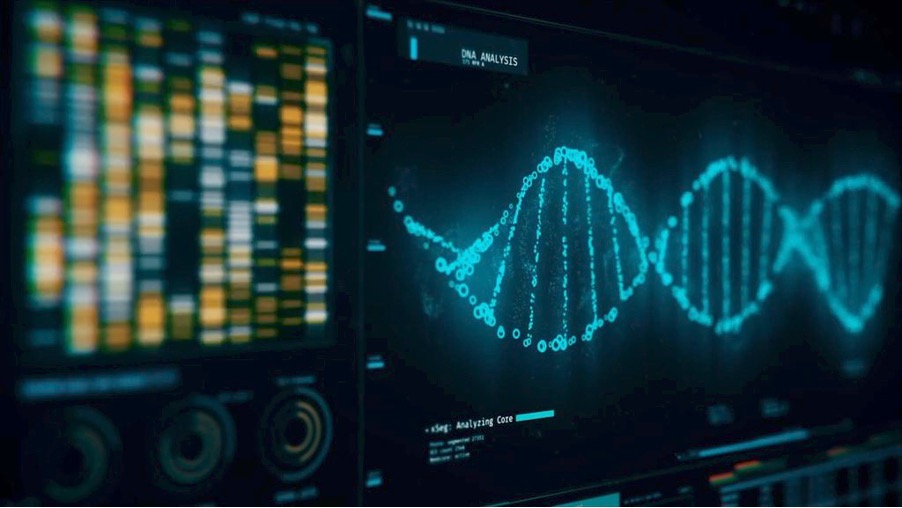
The prominence of computational techniques in the field of research is rising rapidly. (Source:NASA’s Marshall Space Flight Center via Flickr)
COVID-19 seems to have instigated a significant transformation in scientific research methodologies, inaugurating a new age in research that is characterised by computational methods and mathematical models. While the prevalence of computational techniques in research has been on the rise for decades, COVID-19 has helped highlight the exact use and advantage of relying on computational methods, especially in the life sciences.
A chief example of computational methods at work are those employed to help produce vaccines against the coronavirus, SARS-CoV-2. The development of the coronavirus vaccine has been the quickest in the history of modern medicine – in a mere six months after the first COVID-19 case, the vaccine for the infectious disease was already in the last stages of clinical trials (Arnold, 2020). A process that usually takes 20 years was compressed into six months, fast-tracked due to the urgent need for vaccines to control the spread of the disease. The acceleration of vaccine development was assisted by computational immunology. Computational approaches allowed the genomic sequence of the virus to be mapped within weeks of its emergence. By knowing the genomic sequence, researchers could pinpoint the part of the virus that needed to be attacked by the vaccine. With a clearer picture of the viral antigen to target, scientists could make better predictions on viable vaccine candidates.
It is not so surprising that computational methods would be helpful for vaccine development. The human immune system is highly complex, and hence, scientists have turned to mathematical models to “deconstruct” the system and understand it better. The mid-1990s have been proclaimed as the turning point for computational immunology, with the development of innovative methods spurred on by the rise of the HIV/AIDS epidemic that began in the early 1980s. In the mid-1990s, efforts to sequence the HIV virus’s RNA genome and detect variants (just as scientists are now sequencing SARS-CoV-2 for the same purpose) were generating large data sets that were difficult to analyse manually. The same was true of the DNA sequencing results from the ongoing Human Genome Project, which had started in 1990 with the goal of mapping and understanding all human genes (Arnold, 2020). To meet this influx of new data, scientists turned to computational methods to produce better and quicker results. However, at that time, the field was still in the nascent stages, and scientists failed to secure funding as many fellow researchers couldn’t fathom how mathematical models and algorithms could help understand intricate human systems.
One of the first algorithms that showed promise was a simple one developed by Annie De Groot. De Groot, currently the founder and CEO of the biotechnology company, Epivax, was working at the National Institute of Health at the time. The purpose of the algorithm was to understand how T-cells – a class of immune cells that participate in the adaptive immune response – recognise bacteria. T-cells recognize pathogens when certain proteins on the pathogen’s outer surface (the outer bacterial cell membrane, or the viral coat) bind to a class of human proteins, called the MHC class II proteins. After much work on protein sequences from different pathogens, De Groot’s algorithm evolved into a programme that could assess the amino acids sequences of different pathogenic proteins and rank them according to their probability of binding to a given MHC protein. This allowed her to identify proteins that would make the best vaccine candidates for tuberculosis by reducing the number of possible protein epitopes (structural regions of proteins) in the tuberculosis proteome from 1.6 million to a mere 3,000 – a decrease of 99.8% (Arnold, 2020)!
Besides its role in vaccine development, computational techniques have also been a guiding light for drug development. By harnessing the power of bioinformatics to understand the molecular structure of a pathogen – as was the case with coronavirus vaccine development – viable candidates for drugs can be more quickly identified (Muratov et al., 2021). Structure-based drug discovery (SBDD) is a popular technique to assess the best candidate to combat a particular microbe. SBDD is hastened when it is done computationally. In this method, software programmes are used to evaluate the 3D structure of the biological target on a pathogen, also called the ligand, to suggest the top candidates that can effectively dock, or bind to, the ligand. A ligand may be a protein on the outer surface of a bacterial cell, or in the case of the coronavirus, the oft-discussed ‘spike protein’ that facilitates viral entry into human cells. The drug candidates suggested by these computational studies can then be evaluated for their efficacy using experimental techniques. Ultimately, the goal of SBDD is to translate the conclusions from computational investigations to practical experimental steps.
Despite the evolution of computational techniques, their scope is still relatively restricted. There remains a reasonably large gap between what is supposed to work computationally and what works realistically. Mathematical models can only make predictions, but it is up to experiments to validate these predictions. While various bioinformatic and computational techniques have been employed to shortlist potential candidates for drug repurposing and development to treat COVID-19, not all of them have generated positive results. In fact, in 2020, several pre-print servers stopped accepting research manuscripts purely based on computational techniques because they could often not be corroborated by experimental techniques. This reinforced the fact that computational techniques were not a “shortcut” in the process of research. That said, it is evident that life science research will rely more heavily on computational techniques in the future. One must remember that comprehensive research involves a healthy balance between computational and experimental approaches. The secret to acquiring results efficiently and accurately would involve striking the right balance between the two.
References
Carrie Arnold (July 2020). How computational immunology changed the face of COVID-19 vaccine development. Nature Medicine (News Feature).
Muratov et al., (2021). A critical overview of computational approaches employed for COVID-19 drug discovery. Chemical Society Reviews., 2021, 50, 9121-9151.
Related Posts
Failures of Facial Recognition Amid Widespread Masking
Cover Image: Signs similar to this one have become increasingly...
Read MoreHidden Cell-Based HIV Reservoirs Found via Sugar Signatures
Figure 1: SEM image of the human immunodeficiency virus (HIV)...
Read MoreAddison’s Disease: Why Does it Take an Adrenal Crisis for a Correct Diagnosis?
Figure: Blood samples ready to be tested. Addison’s disease is...
Read MoreA New Hybrid Method for Sequencing the Melanoma Genome
Figure 1: Cell culture in a Petri dish Source: Wikimedia...
Read MoreAre We Addicted to Social Media?
Cover Image: In the current age, social media has become...
Read MoreAntimicrobial Resistance in Underprivileged Communities
This publication is in proud partnership with Project UNITY’s Catalyst...
Read MoreRamya Vishwanath