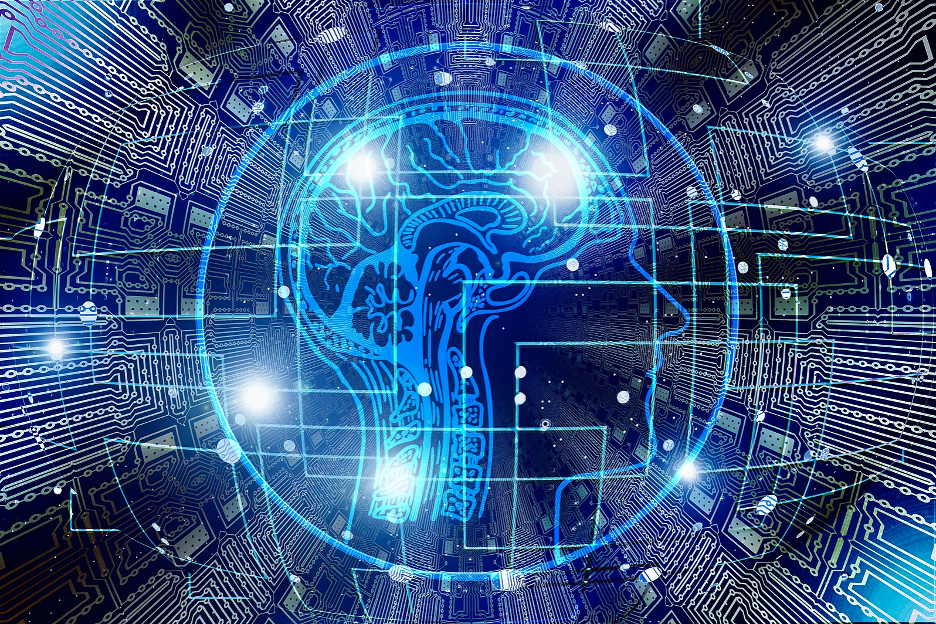
Figure: A colorful display of a stimulated brain surrounded by images representing artificial intelligence. In recent years, the combination of neuroscience and machine learning techniques has given rise to the field of computational neuroscience (Source: Pixabay, Geralt).
The field of computational neuroscience focuses on the use of mathematical models to understand the physiology and cognitive processes of the human brain. This extension of neuroscience allows for a deeper understanding of how the nervous system processes information. Traditionally, computational neuroscience has found its primary applications in the electrical engineering and computer science fields, but recently, it is becoming more prevalent as a tool for the study of human behavior (McDonnell, 2014).
A team of scientists at the University of Helsinki and the University of Copenhagen studying computational neuroscience have developed a technique known as mindful computing to help predict what personal characteristics of others we find physically attractive. The experiment involved scientists placing EEG electrodes onto participants’ heads to test who they found the most attractive among the faces they were shown. The EEG electrodes recorded participants’ brain waves and detected when a participant was more excited or stimulated. This detection system was used as a method of ranking attractiveness and served as a model to gauge attractiveness amongst participants. This model was developed enough so that when a new set of people were shown to the participants (or a new set of participants were shown a set of faces), the scientists were able to predict from past experiments how attractive a participant would find a specific person (University of Copenhagen, 2021).
After producing the model, the scientists executing this experiment did just that – using it to predict which faces a new set of tested participants would find most attractive before they had actually seen them. The findings of this study showed researchers that it is possible to predict what a certain user will personally find attractive, in many different contexts. Streaming services could use this predictive model to personalize TV show recommendations based on its actresses and actors. This model could be especially useful for the ever-growing world of online dating, where online dating companies like Tinder and Bumble could display more accurate potential matches based on a better understanding of users’ preferences (Davidson, 2012). Even restaurant databases such as Yelp and OpenTable could provide recommendations based on an individual’s food preferences as well as the restaurants they frequent the most.
This research falls into the realm of a topic called ‘mindful computing’, a concept that lead author Keith Davis describes as the use of a “combination of computers and neuroscience techniques [by] users […] to access unique information about themselves” (University of Copenhagen, 2021). The emergence of the model described in this study will enable various industries to provide more personalized content to its users, and is expected to be more precise than collaborative filtering. Collaborative filtering is the model used currently by many online industries to tailor pop-up advertisements and posts on social media feeds to a specific individual. Since collaborative filtering relies more heavily on an individual’s choices online, it is a less accurate reflection of their true preferences.
This fact has been made clear by the authors of another scientific review titled “A Survey of Collaborative Filtering Techniques,” written by the Department of Computer Science and Engineering at Florida Atlantic University. Their review discusses the primary shortcoming of collaborative filtering: its dependence on human ratings. Human ratings are often changing and unreliable when forming a predictive model. Collaborative filtering is also unable to group collaborative data and cannot use a collection of data from an individual and their peers to provide exact recommendations. Furthermore, it has been found that societal norms play an important role in an individual’s decisions, so the inability to take others’ opinions into consideration through the collaborative filtering model leads to erroneous recommendations (Su & Khoshgoftaar, 2009).
The concept of mindful computing focuses on taking real-time brain activity from EEG electrodes and translating them into accurate predictions of people’s behavior. Given that this is a newer development in the field of computational neuroscience, it is unlikely that it will be practiced outside of the laboratory for at least another ten years. However, this newer and more precise model of predictions could allow for extremely individualized recommendations. It will be interesting to see the impact of mindful computing within the field of computational neuroscience and without – on social media, e-commerce, and online advertising platforms – in the coming years.
References
- Davidson, A. P. (2012). Collaborative modelling: The future of computational neuroscience? Taylor&Francis Online. https://www.tandfonline.com/doi/citedby/10.3109/0954898X.2012.718482?scroll=top&needAccess=true.
- McDonnell, M. D., Boahen, K., Ijspeert, A., & Sejnowski, T. J. (2014). Engineering Intelligent Electronic Systems Based on Computational Neuroscience. Proceedings of the IEEE. Institute of Electrical and Electronics Engineers, 102(5), 646–651. https://doi.org/10.1109/JPROC.2014.2314776
- Su, X., & Khoshgoftaar, T. M. (2009). A Survey of Collaborative Filtering Techniques. Advances in Artificial Intelligence, 2009, 421425. doi:10.1155/2009/421425
- University of Copenhagen – Faculty of Science. (2021). Computers can now predict our preferences directly from our brain. ScienceDaily. Retrieved June 20, 2021 from www.sciencedaily.com/releases/2021/06/210607110302.htm
Related Posts
The Neuronal Basis for Multitasking
Figure 1: The human brain and its various functional regions. Researchers...
Read MoreVideo Games Shape Childhood Cognitive Development
Source: Caleb Oquendo The first video game was introduced in...
Read MoreFailures of Facial Recognition Amid Widespread Masking
Cover Image: Signs similar to this one have become increasingly...
Read MoreThe Gut May Be The Door to Effective Depression Treatment
First Author: Jillian Troth1 Co-Authors [Alphabetical Order]: Roxanna Attar2, Caroline...
Read MoreBile Acid-Induced Satiety to Treat Obesity
Figure 1: Diagram of the effects of bile acids on...
Read MoreKrithika Nathamuni