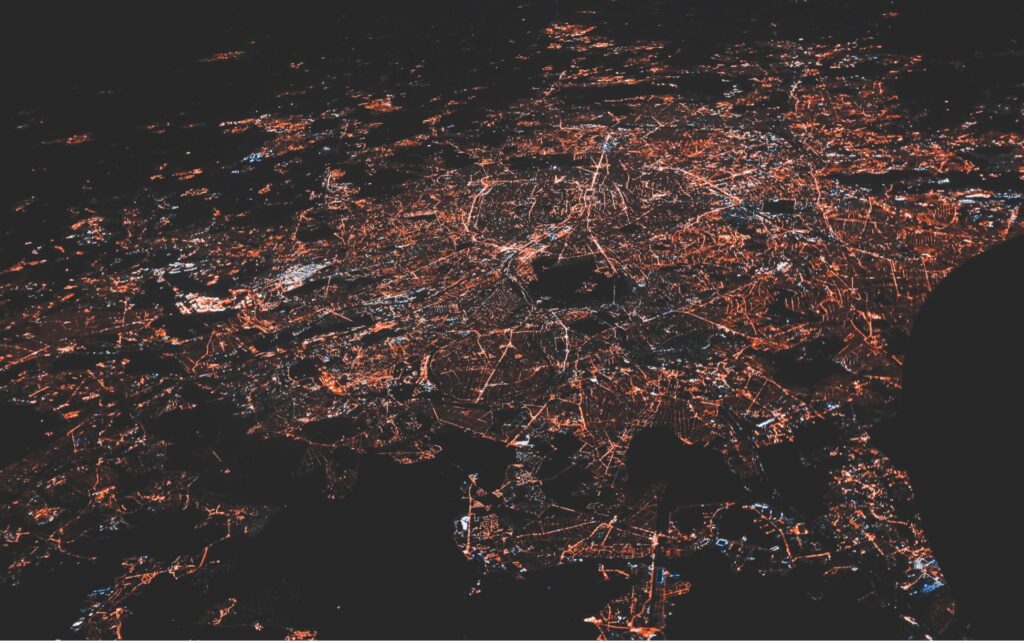
Source: Nastya Dulhiier
How do you treat bacterial infections without causing drug resistance? You might think that targeting the bacterial genes necessary for bacterial growth would be the most effective strategy. However, when you target genes that are essential for growth, bacteria are more likely to develop drug resistance. This is natural selection in action. Bacteria are constantly dividing, multiplying, and mutating, splitting off into many genetically distinct subpopulations (some of which are drug-resistant). When you administer a drug that kills off most of the bacteria, you simply clear the way for drug-resistant subpopulations to multiply and take center stage.
Researchers have come to believe that what we really want to do is target genes that contribute to disease (so-called virulence genes), but that aren’t essential for bacterial growth. In other words, we need drugs that strip bacteria of their disease-causing capabilities without decimating the existing bacterial population and allowing for drug-resistant strains to take over.
For Pseudomonas aeruginosa – a dangerous pathogen that afflicts people with the genetic disease cystic fibrosis (CF) and other immune-compromised conditions – many virulence genes are already known1.But not every virulence gene will do. Because genes operate in complex networks, the expression of one gene can impact the expression of others, either directly or indirectly. Certain virulence genes, while contributing to disease, may also be essential for bacterial growth and survival in the lungs. Targeting them would likely incur drug resistance. The goal, then, is to find genes that contribute to disease, but are not closely tied to bacterial growth.
This is where the featured study comes in. Researchers in the US and Denmark developed a computational model called a genome-scale metabolic network (GEM) to determine the relationship between virulence genes and P. aeruginosa metabolism. The GEM is constructed based on the P. aeruginosa genome – which is why it is called a genome-scale metabolic network. But it also draws on other experimental data collected across many prior studies. The point of the network is to predict how the activity of certain genes will affect bacterial metabolism, and therefore bacterial growth (metabolism, by definition, involves the breakdown of nutrients and construction of key cellular components).
The power of a computational model like this one is that you can predict how knocking out specific genes will impact bacterial growth. And you can do this on a computer (‘in silico’), without performing expensive and time-consuming experiments up front. Ultimately, researchers do want to verify the model’s predictions with actual experiments where specific genes are knocked out. But they can pick specific genes to test in a more educated manner – instead of guessing at which genes would be best to knock out – after the computational model has identified promising targets.
In the featured study, the researchers focused on Pseudomonas genes involved in pyoverdine synthesis. Pyoverdine is a virulence factor that steals iron away from host cells and promotes bacterial infection. Using their model, the researchers were able to identify individual genes in this pyoverdine synthesis gene cluster that – when knocked out – effectively prevent pyoverdine production without dramatically reducing bacterial growth. The pvdA gene stood out in this regard – so the researchers performed an actual experiment to knock it out. They found that knocking out pvdA in P. aeruginosa cells dramatically reduced pyoverdine production, with only a minor reduction in bacterial growth. This makes it a promising therapeutic target.
The model provides useful prediction data on many other virulence genes and gene sets beyond pyoverdine production. Other researchers can test the model’s predictions with laboratory experiments and identify additional viable drug targets. Furthermore, the construction and analysis of metabolic models for other human pathogens will advance drug development efforts across a wide range of diseases.
Featured Publication: Reconstruction of the metabolic network of Pseudomonas aeruginosa to interrogate virulence factor synthesis (Bartell et al., 2017)2
References
- Liao C, Huang X, Wang Q, Yao D, Lu W. Virulence Factors of Pseudomonas Aeruginosa and Antivirulence Strategies to Combat Its Drug Resistance. Front Cell Infect Microbiol 12:926758 (2022). doi:10.3389/fcimb.2022.926758
- Bartell, J., Blazier, A., Yen, P. et al. Reconstruction of the metabolic network of Pseudomonas aeruginosa to interrogate virulence factor synthesis. Nat Commun 8, 14631 (2017). https://doi.org/10.1038/ncomms14631
Related Posts
Gender Differences in Health and Medicine and the Importance of Gender-Specific Medicine in Healthcare Policy
This article was originally submitted to the Modern MD competition...
Read MoreAccording to New Research, Blue Whale Migrations Can Be Studied Through Song
Figure 1: A blue whale’s fluke (tail fin) sticks out...
Read MoreStrength in Numbers: How Community Enhances Perceived Support
Figure 1: Bandmates join in a group hug. The powerful...
Read MoreHow Genetic Engineering Can Responsibly Revolutionize Healthcare Treatment
Source: iStock At just 3 months old, Victoria Gray was...
Read MoreAutism Spectrum Disorder and Gender: The Case for Expanding the Autistic Phenotype
This article was originally submitted to the Modern MD competition...
Read MoreRetitling Stress: A Look at the Yerkes-Dodson Law
Figure 1: This graph compares stress level with performance at...
Read MoreSam Neff