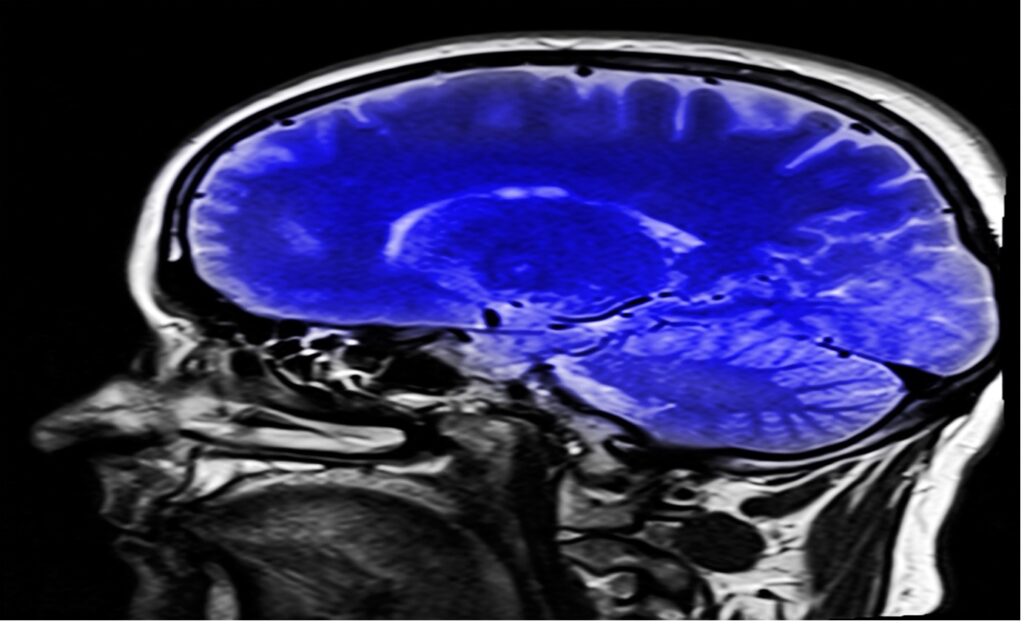
Figure: An image of a Magnetic Resonance Imaging (MRI) scan. Huge advancements in the field of medical physics have made non-invasive monitoring of internal organs such as the brain possible. Recent advancement comes in the form of Magnetometers (MEGs) which offer high spatio-temporal resolution. (Source: Pixabay, Kalhh)
The brain is the most complex part of our body. It is responsible for intelligence, locomotion, memory formation, and many more of our central functions. The human brain is the key to understanding what makes us “human”, what differentiates us from other animals, and what differentiates us from each other. While neuroscientists may be the first researchers who come to mind with this sort of research, learning about the human brain will also lead to advancements in key spheres of Computer Science such as Artificial Intelligence and Machine Learning by motivating the development of new deep learning techniques.
The brain is a part of the central nervous system, and the primary functional unit is the neuron. Much of what we know about the human brain has been discovered by studying neurons, but at the same time, our total knowledge is limited because neuronal interactions are very complex. Truly understanding how the brain works means we need to observe it in real time and determine how its structure changes when exposed to multiple sensory cues (Brain Initiative, n.d.).
Scientists, therefore, need brain imaging techniques that can monitor the brain’s activities. Brain imaging techniques allow scientists to investigate cortical-related anomalies such as Alzheimer’s Disease, Parkinson’s Disease, severe Post-Traumatic Stress Disorder (PTSD), and Autism Spectrum Disorder (Gialopsou et al., 2021; Mandal et al., 2018, Olde Dubbelink et al., 2014, Barik et al., 2020, Dunkley et al., 2020). The list of brain imaging techniques includes Electroencephalography (EEG), functional Magnetic Resonance Imaging (fMRI), Positron Emission Tomography (PET), and Magnetoencephalography (MEG) (Xue et al., 2010; Johns Hopkins Medicine, n.d.; Gialopsou et al., 2021). Some of these techniques even offer the advantage of being non-invasive, meaning the body is not cut open during the imaging process. This non-invasive nature is key because it allows scientists to monitor the functioning of internal organs in real time without disturbing normal physiological function.
When dealing with brain imaging techniques, two measurements are crucial: spatial and temporal resolutions. Spatial resolution refers to the ability to distinguish between two regions of the brain. Temporal resolution refers to the ability to distinguish between two events in the brain taking place at different times (Gialopsou et al., 2021). Spatio-temporal analyses of brain signals are integral to understanding the fundamental mechanisms of neuronal interactions. They also provide reliable biomarkers for discerning physiological and pathological brain activity in neurodegenerative diseases (Gialopsou et al., 2021).
In brain imaging techniques that use magnetism like fMRI, the Biot-Savart law is employed. Electrical currents produce magnetic fields which, depending on the brain imaging technique, can be used to map brain signals (Jog et al., 2016; Hosch, 1998). The Biot Savart law states that the magnetic field due to electric current at any point is inversely proportional to the square of the distance of that point from the current element (Shenoy, n.d.). The consequences of this law are that closer positioning of a sensor system, like the sensors of the fMRI device, provides improved signal-to-noise ratio, better spatial resolution, and more precise source localization (Gialopsou et al., 2021). In other words, brain imaging tools need to be close to the source of the current signals, the human brain.
The most ubiquitous imaging techniques are fMRI and EEG. Blood-Oxygen-Level Dependent (BOLD) fMRI, one variation of the fMRI technique, detects the difference in magnetic field strength between oxygenated hemoglobin and deoxygenated hemoglobin, as the magnetic property of blood depends on its oxygenation level. Cells like neurons require oxygen to carry out their normal activities, and hemoglobin is responsible for transporting oxygen breathed into the lungs to the brain and all other organs. Therefore, scientists can get a sense of neuronal activity indirectly by identifying regions that are more highly oxygenated (regions that possess more oxygenated hemoglobin) (Xue et al., 2010). fMRI maps activated brain regions with high spatial resolution. A major technical challenge for fMRI is that hemodynamic responses are usually very slow, that is, fMRI offers low temporal resolution because the local measured changes in blood flow are not synchronized with neuronal activity (Xue et al., 2010; Gialopsou et al., 2021). To increase the statistical power of fMRI results, many repetitions of the same event are necessary (Xue et al., 2010).
The other major neuroimaging technique, EEG, measures the electrical activities of the brain with electrodes placed on the scalp. EEG is a real-time neuroimaging method with limited source localization capability and spatial resolution, but unlike fMRI, it has a high temporal resolution (Xue et al., 2010; Gialopsou et al., 2021). Scientists would benefit if the two advantages of EEG (relatively high temporal resolution) and fMRI (relatively high spatial resolution) were combined in a brain imaging technique with a high spatio-temporal resolution. Magnetoencephalography (MEG) might be the key.
Like fMRI, MEG is a non-invasive brain imaging technique that measures the magnetic fields generated by electric signals in the brain (Singh, 2014). Unlike fMRI, MEG results have higher temporal resolution and higher spatial resolution (Gialopsou et al., 2021). MEG is usually combined with fMRI to get a good structural perspective of the brain. This combination is called Magnetic Source Imaging (MSI). Some applications of MEG include pre-operative brain mapping for various procedures and epilepsy surgery (Singh, 2014). However, MEG has disadvantages too. Since MEG measures magnetic fields in the range of Femto-Tesla to pico-Tesla (units of magnetic field strength), MEG machines need to be confined in Magnetically Shielded Rooms (MSRs) which subdue environmental magnetic noise (Gialopsou et al., 2021).
Popular types of MEGs include Superconducting Interference Devices (SQUID-MEGs) and Optically Pumped Magnetometers (OPM-MEGs). SQUIDs employ the principles of quantum physics to detect small electrical currents with high sensitivity and a large dynamic range. Thus, SQUIDs when utilized with MEGs, can be used to detect cortical activity. The cortex is the outer layer of the brain responsible for higher-level reasoning and conscious thought – so there is much interest in interrogating its activity. OPM-MEGs are an alternative to SQUID-MEGs (Baillet, 2017; Gialopsou et al., 2021). OPM-MEGs are less expensive and can also be positioned directly on the scalp surface, so they pick up on subtle fluctuations in field strength more effectively. The design of the helmet and sensor head fixes the scalp to sensor distance to approximately 5 mm for OPM-MEGs, while for SQUID-MEGs, it is approximately 50 mm (Gialopsou et al., 2021). With these being the two most popular techniques, there is an ongoing quest to determine whether OPM-MEGs are better scanners when compared to SQUID-MEGs.
Aikaterini Gialopsou and her colleagues from the University of Sussex sought to confirm spatio-temporal measurements of visually evoked fields using OPM-MEGs versus SQUID-MEGs. The experiment was conducted on 3 healthy participants with normal vision, and the two visually stimulating experimental designs included a flash stimulus (FS) and a pattern reversal stimulus (PR). FR, as the name indicates, consisted of short white flashes of a length of 0.08 seconds and the PR involved a black and white checkerboard with the colors reversing at 0.5-second intervals. FS and PR are used to evaluate early visual processing, and to detect abnormalities in the visual pathways. Together they form the model, which the researchers call visually evoked brain fields (VEFs), which allows them to investigate the visual function of the brain.
Even though the team confirmed that both SQUID-MEG and OPM-MEG reproduce characteristic brain signatures from well-established prior clinical research, they did find that OPM-MEG is better than SQUID-MEG in brain signal tracking in both space and time. That is, they confirmed that OPM-MEG has a better spatio-temporal resolution than SQUID-MEG. Specifically, since SQUID sensors from SQUID-MEG are farther away from the scalp than those in OPM-MEG, this widespread position of sensors or electrodes combined with SQUID-MEG’s low spatio-temporal resolution may not be able to record coincident responses from close brain signals (Gialopsou et al., 2021).
In conclusion, the research of Gialopsou et al. offers evidence that OPM-MEGs are better than SQUID-MEGs, and that the former should be utilized in clinics and research facilities. OPM-MEGs are better suited for the investigation of biomarkers that are linked to cortical abnormalities like autism, PTSD, AD, etc., which in turn present themselves in physiological anomalies (Gialopsou et al., 2021). This could help clinicians to detect these diseases early and advise appropriate therapies.
References
Baillet, S. (2017). Magnetoencephalography for brain electrophysiology and imaging. Nature Neuroscience, 20(3), 327–339. https://doi.org/10.1038/nn.4504
Barik, K., Watanabe, K., Bhattacharya, J., & Saha, G. (2020). Classification of Autism in Young Children by Phase Angle Clustering in Magnetoencephalogram Signals. 2020 National Conference on Communications (NCC), 1–6. https://doi.org/10.1109/NCC48643.2020.9056022
Brain Initiative. (n.d.). Why Study the Brain? The BRAIN Initiative. Retrieved December 27, 2021, from https://www.braininitiative.org/why-study-the-brain/
Dunkley, B. T., Jetly, R., Pang, E. W., & Taylor, M. J. (2020). New perspectives on the neurobiology of PTSD: High-resolution imaging of neural circuit (dys)function with magnetoencephalography. Journal of Military, Veteran and Family Health, 6(S1), 16–25. https://doi.org/10.3138/jmvfh.2019-0029
Gialopsou, A., Abel, C., James, T. M., Coussens, T., Bason, M. G., Puddy, R., Di Lorenzo, F., Rolfs, K., Voigt, J., Sander, T., Cercignani, M., & Krüger, P. (2021). Improved spatio-temporal measurements of visually evoked fields using optically-pumped magnetometers. Scientific Reports, 11(1), 22412. https://doi.org/10.1038/s41598-021-01854-7
Hosch, W. (1998, July 20). Biot-Savart law | physics | Britannica [..Com]. Britannica. https://www.britannica.com/science/Biot-Savart-law
Jog, M. V., Smith, R. X., Jann, K., Dunn, W., Lafon, B., Truong, D., Wu, A., Parra, L., Bikson, M., & Wang, D. J. J. (2016). In-vivo Imaging of Magnetic Fields Induced by Transcranial Direct Current Stimulation (tDCS) in Human Brain using MRI. Scientific Reports, 6(1), 34385. https://doi.org/10.1038/srep34385
Johns Hopkins Medicine. (n.d.). Positron Emission Tomography (PET) [..Org]. Johns Hopkins Medicine. Retrieved December 28, 2021, from https://www.hopkinsmedicine.org/health/treatment-tests-and-therapies/positron-emission-tomography-pet
Mandal, P. K., Banerjee, A., Tripathi, M., & Sharma, A. (2018). A Comprehensive Review of Magnetoencephalography (MEG) Studies for Brain Functionality in Healthy Aging and Alzheimer’s Disease (AD). Frontiers in Computational Neuroscience, 12, 60. https://doi.org/10.3389/fncom.2018.00060
Olde Dubbelink, K. T. E., Hillebrand, A., Stoffers, D., Deijen, J. B., Twisk, J. W. R., Stam, C. J., & Berendse, H. W. (2014). Disrupted brain network topology in Parkinson’s disease: A longitudinal magnetoencephalography study. Brain, 137(1), 197–207. https://doi.org/10.1093/brain/awt316
Shenoy, M. (n.d.). Biot Savart law (vector form) (video) | Khan Academy. Retrieved December 28, 2021, from https://www.khanacademy.org/
Singh, S. P. (2014). Magnetoencephalography: Basic principles. Annals of Indian Academy of Neurology, 17(Suppl 1), S107–S112. https://doi.org/10.4103/0972-2327.128676
Xue, G., Chen, C., Lu, Z.-L., & Dong, Q. (2010). Brain Imaging Techniques and Their Applications in Decision-Making Research: Brain Imaging Techniques and Their Applications in Decision-Making Research. Acta Psychologica Sinica, 42(1), 120–137. https://doi.org/10.3724/SP.J.1041.2010.00120
Related Posts
Video Games Shape Childhood Cognitive Development
Source: Caleb Oquendo The first video game was introduced in...
Read MoreHuman Endogenous Retroviruses Might Unlock a New Field of Neurodegenerative Disease Research
Figure 1: Researchers have found viral genetic materials within the...
Read MoreAdvances in the Search for an Alzheimer’s cure
Cover Image: This image displays the differences between a normal...
Read MoreMind Missing Out? Fear of Missing Out and the Brain
Figure: A person scrolling through news articles on their smartphone....
Read MoreEmotion-Related Impulsivity and Disordered Eating Behaviors: A Literature Review
Figure: An artistic depiction of disordered eating behavior under the...
Read MoreAlleviating Vaccine Hesitancy: The Path to a Safer Future
The human mind is constantly working on the fly, adapting...
Read MoreSam Neff