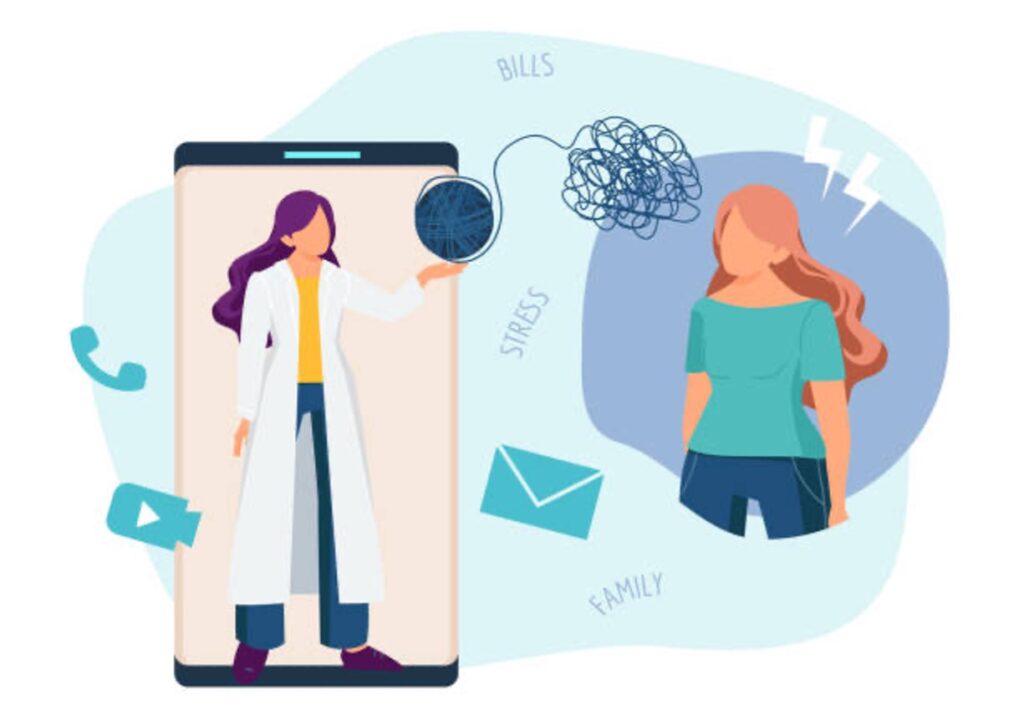
Figure 1: Telehealth consultation via smartphones can serve as a source of at-home mental health care—a service that has become particularly beneficial during the COVID-19 pandemic
Source: Pixabay
The uncertainties caused by COVID-19 could present severe risks of damage to our mental health. These high-risk circumstances include social isolation, widespread unemployment, stress surrounding contracting the virus, social media exposure, and the rising death toll. Global needs for psychological care are increasingly surging, while mandatory restrictions on travel makes it even harder to seek face-to-face mental health care services for people in countries where they are less accessible. This unsatisfying situation calls for re-organization of the continuous delivery of mental health services.
In response to the above challenges, there has emerged an accelerated move in mental health towards the adoption and implementation of digital tools – tele-mental health, that can be offered at a physical distance. Telehealth platforms that utilize a combination of machine learning and sophisticated risk factor algorithms are vital to the delivery of health care services by health care professionals, typically conducted via information and communication technologies such as smartphones or computers. Drawn risk factors from continuously clinical follow-up, tele-health can facilitate the compilation of longitudinal and multivariate datasets from a diverse array of patients. In addition, by recognizing early physical or mental risk markers, tele-health can improve clinical detection and informed prediction, both of which assist tailored prevention. However, some clinical researchers doubted this approach because it obscures some key indicators that can be recognized with an in-person diagnosis procedure, such as physical gestures, and overall appearance. However, existing studies show compelling justification for this internet-based approach using self-reported data. For example, Chandler and Shapiro (2016) suggested that the Amazon Mechanical Turk (MTurk), a popular labor market for crowd-sourcing workers, can obtain samples with psychological symptoms as valid as other diagnostic methods (Mortensen, K. et al., 2018; Kim, H. S. et al., 2017). Comparing data collected via MTurk participants within DSM-5 model to data collected using undergraduate clinical samples, one personality disorder study found convincing consistency (Miller, J. D. et al., 2017).
Two key aspects of the tele-health process can illustrate how tele-health works in practice.
The first aspect is its ability to provide a better understanding of the general population. Common digital data obtained from smartphones can reveal important aspects in our daily life, such as our social engagement, mobility, sleep states, and exercise routines (Cornet & Holden 2018). This data can be derived from accelerometer, global positioning systems (GPS), light sensors, text messaging, emails, calls, app use (e.g., social media use), and microphones or cameras. For example, sleep disturbance, one core diagnostic symptom of depression, in addition to increased patterns of later-to-bed, later-to-rise behavior, can be difficult to measure retrospectively. However, studies using light sensors and phone use data have found sleep disturbance in depression (Ben-Zeev et al. 2015). Severe depression symptoms can also be inferred from reductions in mobility metrics from GPS. Sufferers visit fewer locations, spend more time at home, and move less through geographic space (Ben-Zeev et al. 2015), consistent with the sedentary feature of depression.
Apart from detection and prediction utility, tele-health also plays a promising role in treatment follow-up. One very helpful application is the use of machine learning algorithms to perform hierarchical clustering based on individual self-reported data. One study, clustering individual symptom items from over 7,000 patients with depression, identified three robust symptom clusters that differed in antidepressant response (Chekroud et al. 2017). Selective serotonin reuptake inhibitors (SSRIs), one of the most common antidepressant drugs, can take 4-6 weeks to begin working before a patient has an opportunity to understand the clinical benefit of that drug on their symptoms. Applying machine learning, telehealth (e.g., smartphone-based) research might help reveal the mechanisms of drug action in real-life, longitudinal settings.
Turning to mental health research, our understanding of the neurobiological basis (correlates) of mental illness has fallen short of converting into major advances in detection, prediction, and treatment (Gillan & Rutledge, 2021). A key concern is how we can apply the results of theoretical research in real-life circumstances to help people deal with psychological uncertainty. Fortunately, tele-health enables us to monitor the development of mental diseases. For example, the Sea Hero Quest, an interactive phone game, was designed to develop new diagnostic tools and treatments for dementia. App users chase creatures around a virtual sea to demonstrate their navigation ability, which is measured on an assigned scale.
Despite all these appealing advantages, this internet-based, remote smartphone method of telehealth care is a relatively new area and still faces several unsolved problems regarding privacy, fairness, transparency, and accountability. For example, ethical dilemmas concerning participants’ privacy and anonymity, the gender and/or racial percentage of algorithm designers, and limited access from certain social groups (e.g., elderly or people from disadvantaged backgrounds) all pose challenges to current tele-health techniques.
Telehealth can become a vital component of healthcare for the general population, health care providers, and scientific researchers in preventing, diagnosing, treating, and controlling diseases during the COVID-19 outbreak. Continued flexible funding, administrative support, and instructive guidance might improve the utility of this prospective method during and after the pandemic.
References
Ben-Zeev, D., Scherer, E. A., Wang, R., Xie, H., & Campbell, A. T. (2015). Next-generation psychiatric assessment: Using smartphone sensors to monitor behavior and mental health. Psychiatric Rehabilitation Journal, 38(3), 218-226. http://dx.doi.org/10.1037/prj0000130
Chandler, J., & Shapiro, D. (2016). Conducting clinical research using crowdsourced convenience samples. Annual Review of Clinical Psychology, 12, 53-81. http://dx.doi.org/10.1146/annurev-clinpsy-021815-093623
Cornet, V. P., & Holden, R. J. (2018). Systematic review of smartphone-based passive sensing for health and wellbeing. Journal of Biomedical Informatics, 77, 120–132. https://doi.org/10.1016/j.jbi.2017.12.008
Chekroud, A. M., Gueorguieva, R., Krumholz, H. M., Trivedi, M. H., Krystal, J. H., & McCarthy, G. (2017). Reevaluating the efficacy and predictability of antidepressant treatments: A symptom clustering approach. JAMA Psychiatry, 74(4), 370-378. http://dx.doi.org/10.1001/jamapsychiatry.2017.0025
Gillan, C. M., & Rutledge, R. B. (2021). Smartphones and the Neuroscience of Mental Health. Annual Review of Neuroscience. https://doi.org/10.1146/annurev-neuro-101220-014053
Kim, H. S., & Hodgins, D. C. (2017). Reliability and validity of data obtained from alcohol, cannabis, and gambling populations on Amazon’s Mechanical Turk.Psychology of Addictive Behaviors, 31(1), 85-94. http://dx.doi.org/10.1037/adb0000219
Miller, J. D., Crowe, M., Weiss, B., Maples-Keller, J., & Lynam, D. R. (2017). Using online, crowdsourcing platforms for data collection in personality disorder research: The example of Amazon’s Mechanical Turk. Personality Disorders: Theory, Research, and Treatment, 8(1), 26-34. http://dx.doi.org/10.1037/per0000191
Mortensen, K., & Hughes, T. L. (2018). Comparing Amazon’s Mechanical Turk Platform to Conventional Data Collection Methods in the Health and Medical Research Literature. Journal of General Internal Medicine, 33(4), 533-538. http://dx.doi.org/10.1007/s11606-017-4246-0
Related Posts
An Introduction to the Ebola Virus
Cover Image: Blood sample of a patient infected by the...
Read MoreExploring the Gut-Microbiota Hypothesis of Neurodegenerative Disease Pathogenesis
First Author: Jillian Troth1 Co-Authors [Alphabetical Order]: Caroline Conway1, Allison...
Read MoreMingcong Tang